ai governance
AI Risk Management Framework: A Step-by-Step Guide for 2025
Noel DCosta
- Last Update :
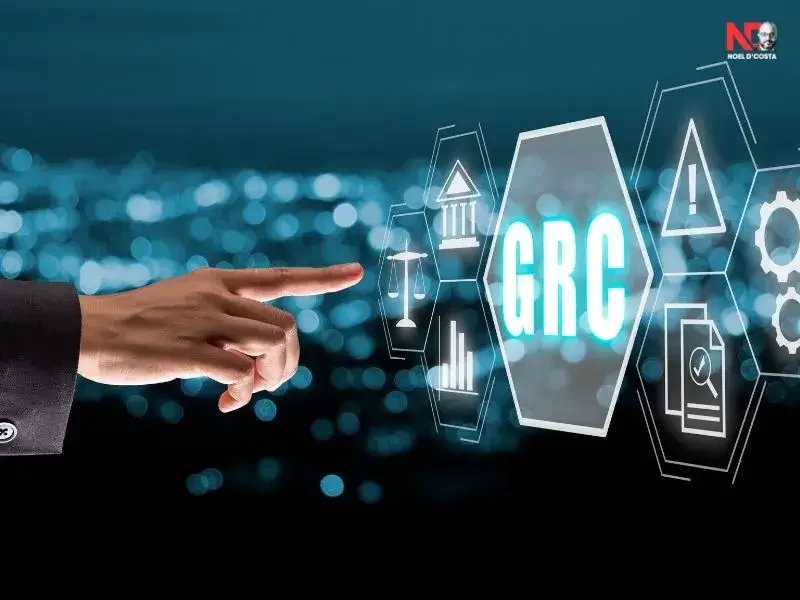
AI is changing the way businesses handle finance, HR, and procurement. It can automate decisions, detect fraud, and streamline operations. But when AI goes wrong, the consequences are serious—bias in hiring, security breaches, regulatory fines, and financial losses. In 2023 alone, AI-related compliance fines exceeded $2 billion, with companies like Clearview AI penalized for data misuse. Without a clear AI Risk Management Framework, businesses risk lawsuits, lost contracts, and public trust.
I’ve seen what happens when AI runs without oversight. A financial institution relied on an AI model for credit approvals, but it unfairly denied loans based on race. The outcome is lawsuits, fines, and a damaged reputation.
Another company automated hiring with AI, only to find it rejecting qualified candidates due to biased training data. AI isn’t foolproof, and without governance, mistakes spiral.
An AI Risk Management Framework helps identify risks, assess their impact, and implement safeguards before issues arise. The goal isn’t to slow AI down—it’s to make sure it operates securely, fairly, and within legal boundaries.
Key areas include:
- Bias Detection: Ensuring AI decisions are fair and explainable.
- Security Controls: Preventing unauthorized access and data leaks.
- Regulatory Compliance: Meeting GDPR, ISO 42001, and AI Act requirements.
- Ongoing Monitoring: Catching issues before they turn into crises.
AI is a powerful tool, but it needs guardrails. A structured AI Risk Management Framework isn’t optional—it’s a necessity. In this guide, I’ll break down the steps to build one that keeps your business safe. Let’s get started.
An AI Risk Management Framework helps organizations identify, assess, and respond to the potential risks that come with deploying AI systems—whether technical, ethical, or operational.It’s less about having all the answers and more about knowing which questions to ask before something breaks or backfires.
What is an AI Risk Management Framework?
An AI Risk Management Framework is, at its core, a way to figure out how to deal with the risks that come with using AI systems. Not just the obvious technical ones—like systems failing or misfiring—but also the subtler stuff. Think bias creeping into decisions, data getting misused, or even outcomes that, well, just don’t make much sense to anyone.
It’s not a checklist, exactly. More like a set of principles or practices that organizations use to ask the right questions. Who’s responsible if something goes wrong? Is the model explainable, or are we just trusting it blindly? Things like that.
Some companies treat it like a compliance issue—they do it because they have to. Others approach it with more caution, maybe because they’ve seen firsthand how things can spiral. I remember reading about a hiring tool that unintentionally filtered out qualified candidates from certain backgrounds. No one had flagged the risk until it was already a problem.
The tricky part is that risks aren’t always easy to spot upfront. And even if you do everything right, something can still go sideways. But that’s kind of the point—the framework exists because certainty isn’t guaranteed. And pretending it is? That’s riskier.
So, let’s talk about what a comprehensive AI Risk Management Framework involves:
Framework Component | Description | Key Actions |
---|---|---|
Governance Structure | Defines oversight bodies and decision rights | Set up AI Ethics Committee; assign Risk Officers |
Risk Identification | Detects potential technical, ethical, and regulatory risks | Perform risk workshops, threat modeling, scenario analysis |
Compliance Alignment | Ensures AI systems comply with laws (e.g., EU AI Act, GDPR) | Map AI use cases to regulatory checklists and compliance audits |
Bias and Fairness Mitigation | Addresses discrimination or inequality in AI outcomes | Conduct bias testing, fairness audits, and demographic impact assessments |
Explainability & Transparency | Makes AI decisions understandable to users and stakeholders | Implement explainable AI (XAI) methods and model documentation |
Security & Privacy Controls | Safeguards AI models and data against attacks | Encrypt sensitive training data; test for adversarial vulnerabilities |
Continuous Monitoring | Tracks AI model behavior post-deployment | Set up KPIs, anomaly detection, and human review loops |
Incident Response | Prepares for AI malfunctions or ethical breaches | Create escalation paths and AI-specific disaster recovery plans |
Stakeholder Communication | Ensures internal and external transparency about AI use | Publish risk disclosures, model cards, and stakeholder updates |
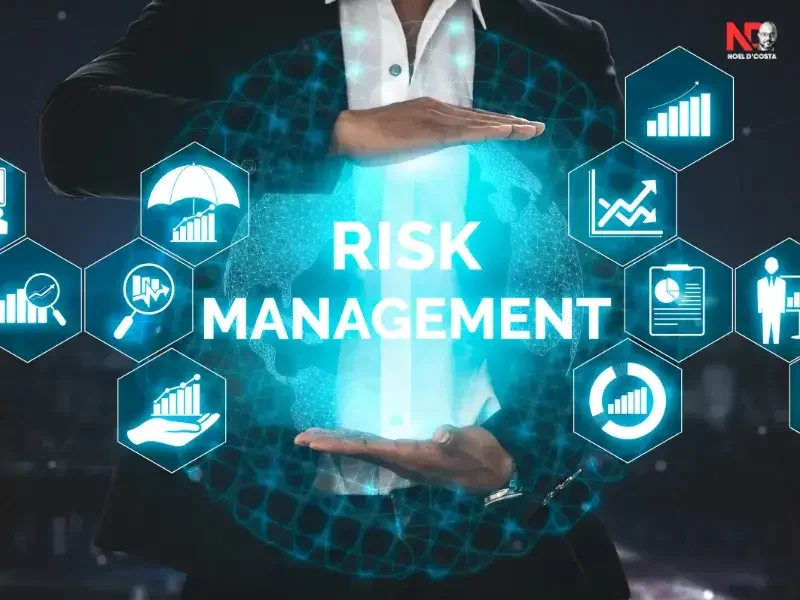
Step | Phase | Key Activity | Outcome |
---|---|---|---|
1 | AI Use Case Inventory | Identify and categorize all AI applications | Clear visibility into AI risk exposure |
2 | Risk Assessment | Conduct technical, ethical, legal risk analysis | Risk severity scoring and prioritization |
3 | Governance Design | Establish AI policies and assign risk owners | Clear accountability and fast decisions |
4 | Compliance Mapping | Align AI systems with regulations | Compliance gap analysis and action plans |
5 | Model Validation | Bias testing, robustness checks, explainability reviews | Fair, safe, and controllable models |
6 | Security Hardening | Apply AI cybersecurity defenses | Resilient AI systems against attacks |
7 | Monitoring Setup | Deploy live monitoring tools for drift and anomalies | Early detection of AI misbehavior |
8 | Incident Management Plan | Create AI-specific incident response procedures | Minimized operational, reputational risks |
9 | Stakeholder Communication | Publish model cards, impact statements | Transparency builds trust and compliance |
10 | Continuous Improvement | Adapt to new risks, update policies regularly | Future-proof, sustainable AI governance |
Step 1: AI Risk Management Framework: Identifying your AI Risks
AI is making big decisions in hiring, finance, and security. When it works, it’s efficient. When it doesn’t, it’s a mess. In 2023, a healthcare AI favored white patients over Black patients, limiting access to care. That wasn’t an accident. It was bad training data, and it led to public outrage, lawsuits, and regulatory scrutiny.
I’ve seen companies ignore AI risks until it’s too late. One had a chatbot that leaked customer data. Another automated hiring and only promoted men. Fixing the damage cost them more than preventing it would have. Spotting AI risks early isn’t optional—it’s a survival strategy.
Common AI Risks
- Bias in Decisions → AI picks up bad habits from bad data. If your hiring AI always picks men for leadership, it’s not being “smart”—it’s being biased.
- Security Vulnerabilities → AI systems are hacker magnets. IBM reported a 74% spike in AI cyberattacks in 2023.
- Compliance Failures → GDPR, the AI Act, and ISO 42001 all have strict rules. Break them, and expect fines, lawsuits, and audits.
- Operational Disasters → AI can automate mistakes at scale. If an AI miscalculates demand, you’ll have empty shelves or overflowing warehouses.
- Public Trust & Ethics → People don’t like AI overreach. That’s why facial recognition is getting banned in cities worldwide.
How to Catch AI Risks Early
- Audit AI models. Don’t assume AI is working correctly—check it.
- Fix your data. Garbage in, garbage out. Bad data = bad AI.
- Test for bias. AI should work for everyone, not just a select few.
I’ve worked with businesses that learned this the hard way. A structured risk plan doesn’t slow AI down—it keeps it from wrecking your business.
Risk Category | Description |
---|---|
Bias & Discrimination Risk | Unfair outcomes due to skewed training data, flawed logic, or underrepresented groups |
Explainability Risk | Inability of end users or auditors to understand how AI systems make decisions |
Security Risk | AI models exposed to adversarial attacks, data poisoning, or model theft |
Privacy Risk | Unauthorized use, leakage, or inference of personal or sensitive data |
Regulatory & Compliance Risk | Violation of legal requirements like GDPR, EU AI Act, HIPAA, or sector-specific rules |
Operational Risk | Failures during deployment, monitoring, or updates that affect business continuity |
Reputational Risk | Public or stakeholder backlash due to unethical or harmful AI behavior |
Data Quality Risk | Model degradation or flawed outcomes due to incomplete, noisy, or outdated data |
Overfitting & Model Generalization Risk | AI performs well in training but poorly in real-world, unseen data |
Automation Risk | Misuse or over-reliance on AI in critical decisions without human oversight |
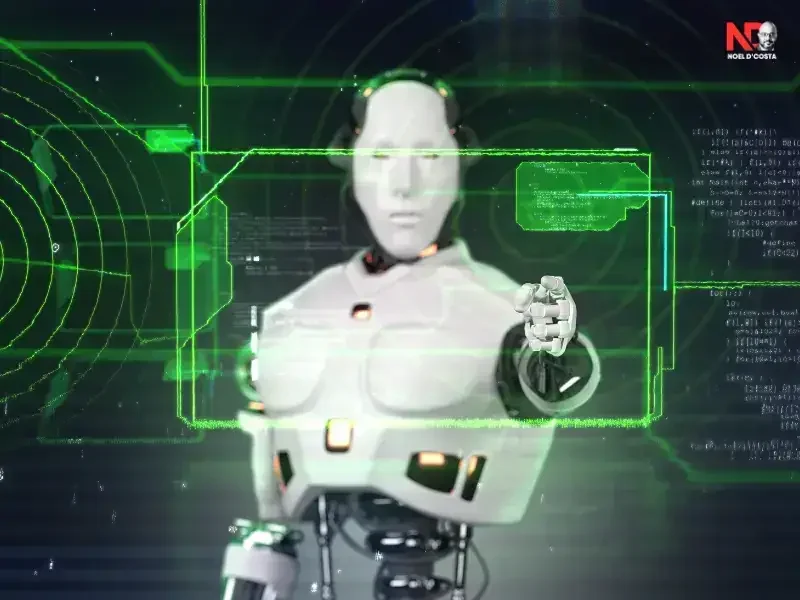
Step 2: AI Risk Management Framework: Risk Assessment & Categorization
Spotting risks is one thing. Knowing which ones can destroy your business is another. AI failures don’t happen in a vacuum. Some are annoying but manageable—others lead to fines, lawsuits, and PR disasters.
Since 2023, many companies got caught using biased algorithms, exposing private data, and violating regulations. I’ve worked with some teams that brushed off AI flaws as “minor issues.” Then those issues went public. The only thing I can tell you about this was that it didn’t end well.
How Do You Know Which AI Risks to Fix First?
Not every AI issue is an emergency. That’s why businesses use risk scoring models. These models rank risks based on two simple questions:
- How likely is this to happen?
- How bad would it be if it did?
How does AI Fail (and Why They Matter)?
- Biased Hiring AI → If someone notices, expect lawsuits. If not, it quietly skews hiring and hurts diversity.
- Fraud Detection False Positives → Stopping legit transactions costs millions and frustrates customers.
- Chatbot Giving Wrong Info → In casual use? No big deal. In healthcare or finance? Huge liability.
What Businesses Do About It
Companies score risks from low to critical and focus on the biggest threats first.
- High likelihood + high impact? Fix it now.
- Low likelihood + low impact? Monitor it.
Ignoring AI risks doesn’t make them go away. You need to manage the risks better. I have written an article “Project Risk Assessment: Prevent Disaster With This Guide“.
Use the same methodology to perform your own Risk Assessment.
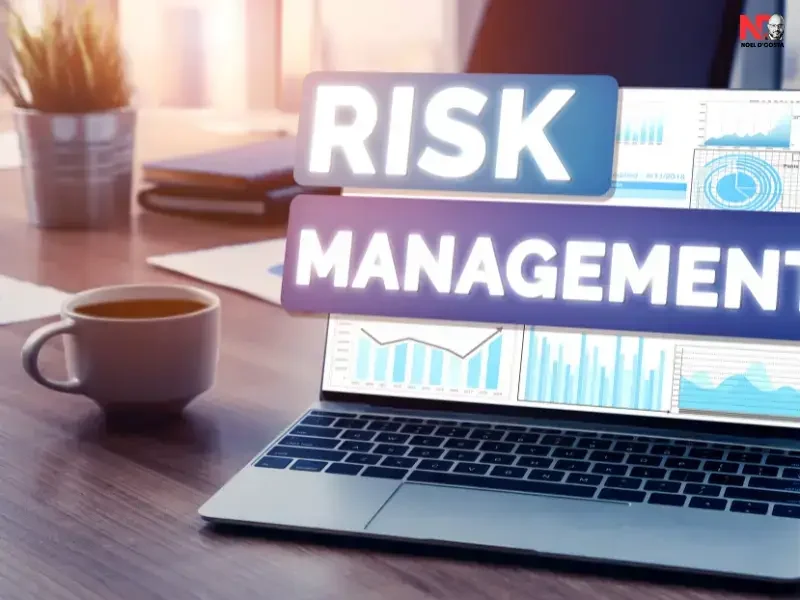
1. AI Risk Heat Maps: Focus on What Actually Matters
Not all AI issues are equal. Some can ruin your business. Others are just annoying. A risk heat map helps teams see which issues need fixing now and which ones can wait.
How Does it Work?
A Traffic light approach would work perfectly for AI Risks:
🔴 Red Zone – Critical Risks
- AI handling money, healthcare, or legal decisions.
- If it screws up, expect lawsuits, fines, or financial disaster.
- Example: AI denies a mortgage by mistake or misdiagnoses a patient. That’s not just a glitch—it’s a crisis.
🟡 Yellow Zone – Moderate Risks
- AI that assists but doesn’t make final decisions.
- If it messes up, it’s annoying, but not catastrophic.
- Example: A chatbot suggests a terrible investment—confusing, but no one’s emptying their bank account over it.
🟢 Green Zone – Low Risks
- AI handling minor, non-sensitive tasks.
- Mistakes here are irritating, not business-ending.
- Example: AI recommends the wrong Netflix show. You’ll live.
Why It Works
I’ve seen companies waste time fixing minor AI issues while ignoring the real threats. A heat map keeps priorities straight.
- 🔴 Fix Red Zone risks ASAP.
- 🟡 Keep an eye on Yellow Zone risks.
- 🟢 Don’t panic over Green Zone risks.
The goal isn’t fix everything—it’s fix what can actually hurt you first.
2. Compliance Risk Matrix: Staying with the Legal Framework
Since 2023, AI laws are being approved in multiple countries. GDPR, the AI Act, HIPAA—regulators are getting stricer. If your AI handles personal data, financial transactions, or hiring decisions, you have to ensure that it’s playing by the rules.
Where AI Can Get You in Trouble
AI Handling Personal Data? → GDPR applies.
- If this is not managed well, you could be paying €20 million or 4% of global revenue.
- Example: Your AI collects customer info without consent? That’s a GDPR fine waiting to happen.
AI in Healthcare? → HIPAA has strict rules on patient data.
- Violations mean up to $1.5 million in penalties—per violation.
- Example: AI shares private medical records where it shouldn’t? Get ready for a lawsuit.
AI Making Hiring or Lending Decisions? → The AI Act cracks down on bias.
- If AI makes unfair decisions, expect lawsuits, PR disasters, and heavy fines.
- Example: AI rejects job applicants because of race, gender, or zip code? That’s discrimination—and it’s illegal.
Why Compliance Risk Matters
I’ve seen companies treat compliance like a checklist—until they had to shut down operations overnight. The cost of ignoring compliance is always higher than fixing it early.
A compliance risk matrix helps spot red flags before regulators do. Stay ahead, fix issues early, and keep AI working for you—not against you.
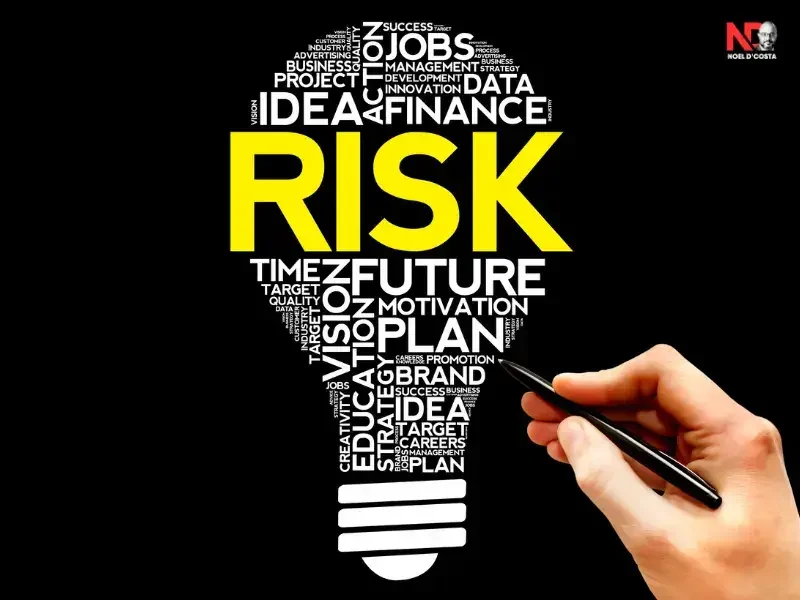
Risk Category | Risk Description | Impact | Likelihood | Risk Rating |
---|---|---|---|---|
Bias & Fairness | Models exhibit unfair or discriminatory results across groups | High | High | Critical |
Explainability | Decisions are not understandable by users or auditors | Medium | High | High |
Data Privacy | Exposure of sensitive or personal data through model inference | High | Medium | High |
Model Drift | Performance degrades due to data changes over time | Medium | High | High |
Adversarial Attacks | Models manipulated via malicious inputs | High | Low | Medium |
Overfitting | Model performs well in training but fails in real data | Medium | Medium | Medium |
Compliance | AI violates GDPR, HIPAA, or AI Act requirements | High | Medium | High |
Operational | System failure in real-time decision scenarios | High | Low | Medium |
Reputational | Negative public or media reaction to harmful AI behavior | High | Medium | High |
Automation Misuse | Critical tasks fully automated without human oversight | Critical | Low | High |
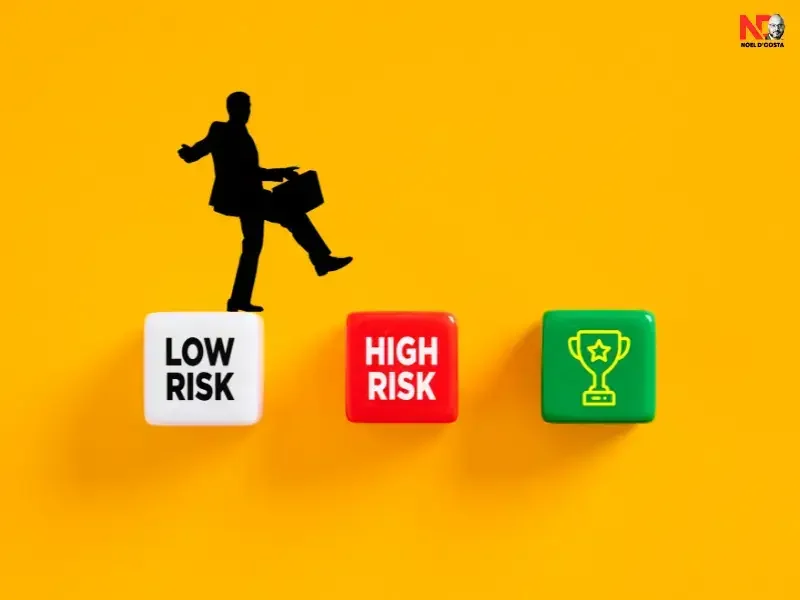
Step 3: AI Risk Management Framework: AI Risk Mitigation Strategies
AI Risks Don’t Fix Themselves
Bias can go undetected for months. Security breaches happen in seconds. I’ve seen companies lose money, customers, and trust because they assumed their AI was working fine. It wasn’t. AI needs constant oversight, testing, and adjustments to stay reliable.
Bias & Fairness: Fix It Before It Wrecks You
AI models inherit bias from data. If your training data is flawed, your AI will be too. Companies have been sued for AI hiring tools that penalized women and lending models that favored high-income borrowers.
How to fix it:
- Fairness-aware algorithms → Adjust models to detect and correct bias.
- Regular bias audits → Check training data to ensure fair treatment.
Amazon scrapped its AI hiring tool when it realized the system was rejecting resumes with the word “women’s.” Ignoring bias isn’t an option.
Security & Privacy: Keep Hackers Out
AI is a prime target for cyberattacks. IBM reported a 74% increase in AI-related breaches in 2023. Hackers can poison training data, manipulate results, or steal sensitive information.
How to fix it:
- Encrypt and anonymize data → Keep personal info secure.
- Adversarial robustness → Protect AI from manipulation.
If AI handles financial transactions, healthcare records, or government data, strong security measures aren’t optional.
Operational Risks: Who’s in Charge When AI Fails?
When AI makes a bad call, who takes responsibility? Businesses need:
- AI explainability tools → Make decisions transparent and justifiable.
- Human-in-the-loop (HITL) → Keep people involved in high-stakes AI decisions.
I’ve worked with companies that only realized AI risks after lawsuits, lost customers, or regulator crackdowns. Managing AI risk isn’t about compliance—it’s about avoiding disasters before they happen.
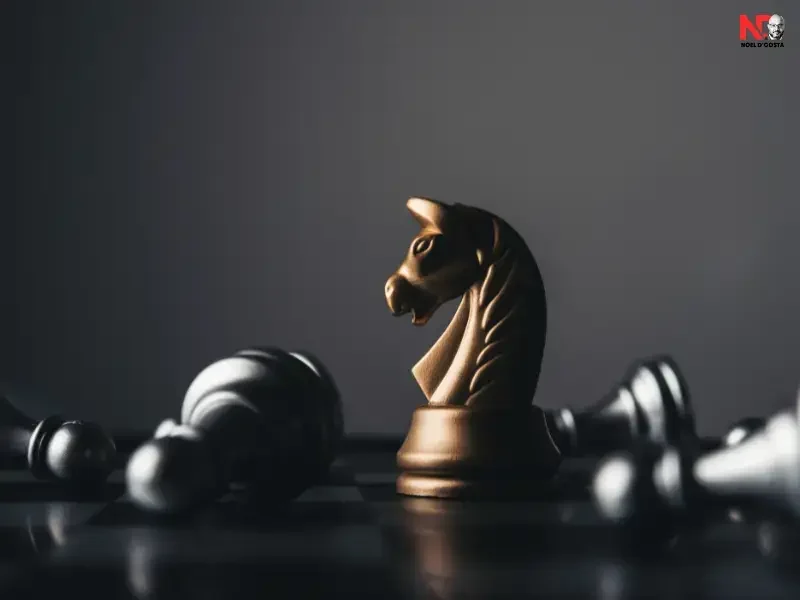
Step 4: AI Risk Management Framework: Implementing AI Governance & Compliance
AI Needs Oversight—Or It’ll Cost You Your Reputation and Money
AI can’t be left to run wild. When models make bad calls, who’s responsible? In 2023, a major bank was fined $30 million when its AI discriminated against minority applicants. They didn’t set up proper governance, and it cost them—big.
I’ve seen companies rush AI into production without thinking about governance. Then, when regulators came knocking or customers complained, they had no answers. That’s why AI needs rules, oversight, and accountability—before things go wrong.
What AI Governance Looks Like
A strong AI governance framework keeps AI transparent, accountable, and compliant.
- Set Up an AI Risk & Ethics Committee → This isn’t a check-the-box task. You need a team that monitors AI risks, enforces ethical guidelines, and keeps AI in line with laws and company values.
- Track AI Decisions with Audit Trails → If AI denies a loan or rejects a job applicant, there should be a clear, explainable reason—backed by data. No black-box excuses.
Avoid Compliance Nightmares
AI regulations are getting stricter, and ignoring them is a financial risk.
- Follow ISO 42001, NIST AI RMF, and GDPR → These frameworks set the standard for AI accountability, privacy, and risk management.
- Audit AI Systems Regularly → AI compliance isn’t a one-time check. Regular reviews catch issues before regulators do.
I’ve seen companies get blindsided by regulators flagging their AI for bias, privacy violations, or security risks. Strong AI governance prevents these surprises—and protects your business from costly mistakes.
Compliance Area | Best Practice | Purpose |
---|---|---|
Data Privacy | Apply GDPR principles (lawfulness, consent, minimization, rights) | Ensure user data is processed legally and ethically |
Bias Mitigation | Test models for statistical parity, fairness, and disparate impact | Prevent discriminatory outcomes and legal exposure |
Transparency | Disclose AI usage and capabilities to end-users and regulators | Enable informed user consent and regulatory review |
Explainability | Deploy XAI tools to interpret decision logic | Support auditability and accountability for AI outputs |
Security | Implement encryption, access control, and adversarial defense | Protect model integrity and data from unauthorized access |
Human Oversight | Define human-in-the-loop controls for critical AI decisions | Maintain accountability and avoid automated harm |
Model Documentation | Maintain detailed model cards and audit trails | Support traceability and compliance evidence |
Risk Classification | Map AI use cases to EU AI Act risk tiers | Determine legal obligations by system risk level |
Incident Management | Establish procedures for AI failure detection and response | Reduce liability and mitigate systemic risk |
Third-Party Vendor Management | Audit AI partners for policy alignment and compliance guarantees | Avoid liability from non-compliant external systems |
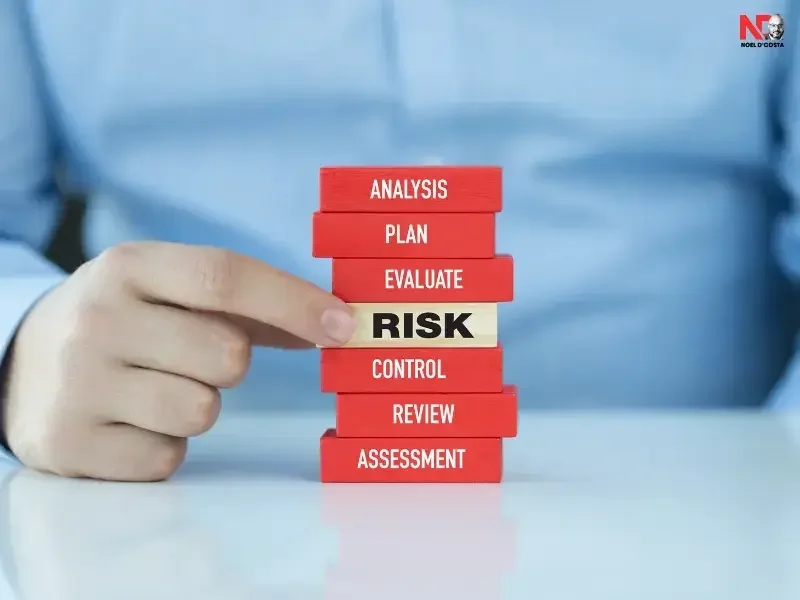
Step 5: AI Risk Management Framework: Continuous AI Risk Monitoring & Incident Response
AI doesn’t flash a warning sign when it goes offcourse. A model drifts, a system misfires, and before anyone notices—customers pay the price.
In 2023, an AI-driven trading algorithm lost $440 million in minutes. No one was monitoring it properly. The system made bad trades, and by the time humans stepped in, it was too late. AI needs real-time monitoring—period.
I’ve seen companies assume their AI is running fine, only to scramble when a silent error snowballs into a crisis. If you’re relying on AI, you can’t afford to react late.
AI Risk Monitoring: What Smart Companies Do
Real-Time Anomaly Detection
- AI models change over time. Some changes are fine. Others? Red flags.
- Monitoring tools catch weird patterns and trigger alerts before bad decisions impact customers.
- Example: A loan approval AI suddenly starts rejecting all applicants from a specific city. If no one’s watching, that’s a lawsuit waiting to happen.
Automated Dashboards
- AI generates tons of data—you need a way to track trends and spot risks fast.
- Dashboards show performance shifts, security issues, and high-risk anomalies in real time.
- Example: A fraud detection AI suddenly blocks too many legit transactions. If you catch it early, you save millions in lost revenue.
Why This Matters
I’ve worked with companies that only realized AI was failing after customers started complaining or regulators started investigating. That’s not a strategy—it’s a liability.
AI risk monitoring isn’t just about tracking performance—it’s about preventing disasters before they happen.
Tool Name | Purpose | Risk Area Covered |
---|---|---|
WhyLabs | Monitors ML model health, data drift, and anomalies | Data Drift, Model Performance, Outliers |
Fiddler AI | Bias detection, explainability, and real-time model monitoring | Bias, Explainability, Compliance |
Arthur AI | Tracks fairness, drift, and performance degradation in models | Fairness, Drift, Accuracy |
IBM Watson OpenScale | Explains decisions and detects bias across deployed AI | Explainability, Bias, Compliance |
Truera | Provides model insights, debugging, and fairness testing | Model Debugging, Fairness |
Robust Intelligence | Automatically stress-tests AI models for edge case failure | Robustness, Adversarial Vulnerability |
Credo AI | Governance platform for policy enforcement and compliance checks | Policy Compliance, Documentation |
Microsoft Responsible AI Dashboard | Monitors fairness, error analysis, and data imbalance | Fairness, Performance, Bias |
Seldon Deploy | Production-grade deployment with model drift and performance alerts | Drift, Production Monitoring |
Amazon SageMaker Clarify | Detects bias in training and inference data | Bias Detection, Feature Attribution |
Incident Response: What You Do When AI Fails
Even with the best monitoring, AI will fail at some point. What separates companies that recover quickly from those that spiral into chaos is a clear response plan.
I’ve seen teams scramble when AI failures caught them off guard. No one knew who to alert, how to stop the damage, or what to tell customers. By the time they figured it out, the problem had escalated.
How to Handle AI Failures
Predefined Escalation Procedures
- When AI messes up, who gets the call? It should be clear who is in-charge.
- What steps should be followed immediately to manage this situation?
- Example: A fraud detection AI starts flagging every transaction as suspicious. Do you have a team ready to step in fast?
Crisis Management for AI-Driven Failures
- AI failures can hit customers, finances, and compliance all at once.
- A clear and tested plan ensures quick damage control, clear communication, and fast system recovery.
- Example: An AI-driven chatbot leaks sensitive user data. Without a response plan, you’re dealing with lawsuits, lost trust, and PR fallout.
Why This Matters
I’ve personally watched businesses lose customers and damage their reputation just because they weren’t prepared for AI failures.
Besides protecting business operatioins, a strong incident response plan protects reputations. The faster you respond, the less damage AI failures cause.
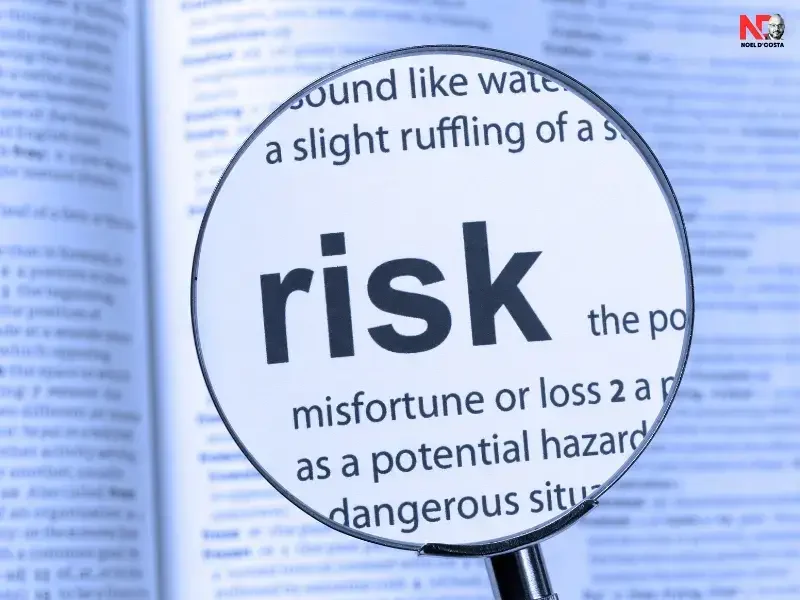
Case Study: AI Risk Management in Action
In 2018, Amazon had to scrap its AI hiring tool after realizing it discriminated against women. The system was trained on ten years of past resumes—most from men. The result was that it penalized resumes with words like “women’s” and favored traditionally male-associated terms.
An AI meant to make hiring fairer and faster ended up reinforcing gender bias. A failure like this wasn’t a glitch—it was predictable and preventable with proper risk management.
How AI Risk Management Could Have Prevented It
This wasn’t a sudden breakdown—the bias was baked into the data. A detailed AI risk management framework would have caught it early by:
- Running Bias Audits → Regular testing of AI decisions would have flagged the skewed results.
- Ensuring Fair Training Data → A more balanced dataset would have prevented the model from learning biased patterns.
- Keeping Human Oversight → AI should support decisions, not replace humans. Recruiters in the loop could have stopped bad calls.
Lessons Learned: What Companies Do Now
Amazon’s failure became a warning sign for businesses using AI in hiring, lending, and healthcare. Now, smart companies:
- Use Bias Detection Tools → AI models get audited to spot discrimination early.
- Make AI Explainable → Hiring tools must show why they pick or reject candidates.
- Follow Equal Opportunity Laws → AI decisions must be fair—or risk legal trouble.
I’ve seen companies fix AI bias before it causes harm—but only when they invest in risk management from day one. I’m sure you know that AI doesn’t fix itself. The earlier you catch risks, the easier they are to control.
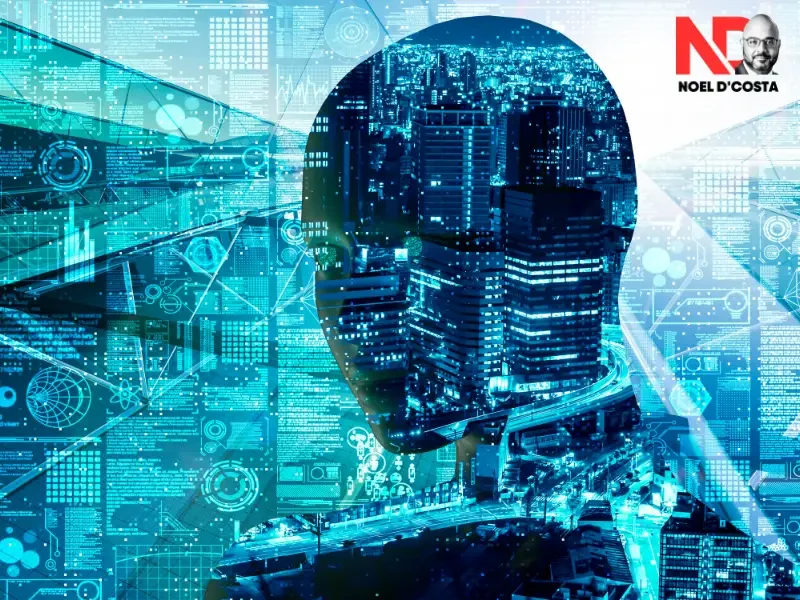
Conclusion
AI can do a lot of good, if it is managed in the right way. I hope I have been able to cover all the key areas of AI Risk Management Frameworks and it’s importance.
A hiring model rejects qualified candidates because of biased training data. A fraud detection system blocks legit transactions and costs millions in lost revenue. A chatbot accidentally leaks personal info.
These aren’t hypothetical issues. They’re happening right now.
I’ve seen companies ignore AI risks because they assumed “it’s working fine.” Then one day, regulators showed up, customers complained, or a security breach hit the headlines.
Smart businesses don’t wait for disaster. They:
- Check for bias before it causes legal trouble.
- Monitor your AI related systems in real time to catch failures early.
- Have a plan in place for when things go wrong.
The companies that take AI risk management seriously today are the ones that won’t be scrambling tomorrow.
Now, I want to hear from you. Is your business prepared for AI risks? Have you faced challenges with bias, compliance, or security? Drop a comment and let’s talk.
If you have any questions or want to discuss a situation you have in your AI or ERP journey, please don't hesitate to reach out!
Frequently Asked Questions
1. What is AI Risk Management?
AI risk management is the process of identifying, assessing, and mitigating risks associated with artificial intelligence. AI systems can introduce bias, security vulnerabilities, compliance violations, and operational failures, which can lead to financial loss, legal issues, or reputational damage.
A structured risk management approach helps businesses:
- Ensure fairness by reducing bias in AI decision-making.
- Secure AI systems from cyber threats, data breaches, and adversarial attacks.
- Stay compliant with global regulations like GDPR, HIPAA, and the AI Act.
- Prevent operational failures that could disrupt business processes.
Without AI risk management, organizations are exposed to uncontrolled AI behaviors, regulatory fines, and ethical concerns that can severely impact business continuity.
2. Why is AI Risk Management Important?
AI is a powerful tool, but when used without proper oversight, it can amplify risks instead of solving problems. Consider these real-world examples:
- Amazon’s AI-powered hiring tool was scrapped after it was found to favor male candidates over women.
- AI fraud detection systems have incorrectly flagged legitimate transactions, causing business losses and customer frustration.
- Chatbots and AI-generated content have spread misinformation, damaging brand credibility.
By implementing AI risk management, companies can:
- Avoid regulatory penalties and lawsuits caused by non-compliance.
- Improve AI accuracy and reliability to prevent costly errors.
- Ensure public trust by making AI systems transparent and fair.
Organizations using AI in critical operations—like finance, healthcare, and HR—must prioritize risk management to protect both their business and their customers.
3. What Are the Biggest Risks in AI?
AI risks vary by industry, but the most common include:
- Bias & Fairness Risks – AI models trained on biased data can discriminate based on race, gender, or socioeconomic status.
- Security Risks – AI systems can be hacked, data can be poisoned, and adversarial attacks can manipulate AI outputs.
- Compliance & Regulatory Risks – AI that violates GDPR, the AI Act, or HIPAA can result in hefty fines and legal action.
- Operational Risks – AI failures can disrupt supply chains, automate errors at scale, or make incorrect financial decisions.
- Ethical & Societal Risks – AI decisions impact fairness, trust, and human rights. For example, facial recognition bans in cities worldwide show the public won’t accept AI overreach.
By proactively managing these risks, businesses can avoid legal, financial, and reputational damage.
4. How Can Companies Identify AI Risks?
AI risks can be subtle, but companies can catch them early using:
- AI Model Audits – Regularly review how AI makes decisions to detect issues.
- Bias & Fairness Evaluations – Test AI models across different demographic groups to ensure equal treatment.
- Data Quality Assessments – Verify training data is accurate, representative, and free from hidden biases.
- Security Vulnerability Tests – Simulate cyberattacks to check if AI systems can be compromised.
Identifying AI ris
5. How Can Businesses Reduce AI Bias?
AI bias can lead to discrimination, lawsuits, and loss of public trust. Companies can reduce bias by:
- Using diverse training data – Ensure AI models are trained on representative data covering all user demographics.
- Implementing fairness-aware machine learning techniques – Use algorithms designed to detect and minimize bias.
- Regular bias audits – Continuously test AI systems for unintended discrimination.
- Introducing human oversight – Keep humans involved in critical AI decisions, especially in hiring, lending, and law enforcement applications.
Ignoring bias can result in public backlash and legal consequences, making it crucial to address fairness issues proactively.
6. What Are Some AI Security Risks?
AI systems face growing security threats as hackers develop new ways to exploit them. Some of the biggest security risks include:
- Adversarial Attacks – Hackers manipulate AI inputs to trick models into incorrect decisions (e.g., altering images to fool facial recognition).
- Data Poisoning – Malicious actors insert corrupted data into AI training sets to compromise AI outputs.
- Unauthorized Access – Weak security measures allow hackers to steal sensitive AI-generated data.
- AI Model Theft – Cybercriminals attempt to copy proprietary AI models and use them without authorization.
To protect AI systems, companies should use encryption, anomaly detection, and adversarial defense techniques.
7. How Does AI Risk Management Align With Compliance?
AI risk management is directly tied to compliance, helping businesses meet legal and ethical standards. Some key regulations include:
- GDPR & CCPA – Protect personal data in AI models and ensure users can opt out of automated decisions.
- AI Act (EU) – Enforce transparency and accountability for AI-driven decisions.
- ISO 42001 & NIST AI RMF – Provide global standards for responsible AI governance.
- HIPAA (Healthcare AI Compliance) – Protects patient data used in AI healthcare applications.
By embedding compliance into AI risk management strategies, businesses avoid penalties, maintain public trust, and build AI systems that meet legal requirements.
8. What Are the Best Practices for AI Risk Management?
Managing AI risks requires a proactive approach. The best practices include:
- Implementing AI Risk Monitoring Tools – Use real-time dashboards to track AI model performance and detect issues before they escalate.
- Regular AI Model Audits – Conduct routine reviews to ensure accuracy, fairness, and compliance.
- Establishing Human Oversight – Keep humans in the loop for high-risk AI decisions, such as hiring and financial approvals.
- Developing an AI Incident Response Plan – Have a predefined strategy for handling AI failures, security breaches, or ethical concerns.
- Training Employees on AI Risks – Educate teams on AI compliance, bias prevention, and cybersecurity.
AI risk management isn’t one-and-done—it’s an ongoing process that evolves with technology and regulations.
9. What is the NIST AI Risk Management Framework?
The NIST AI Risk Management Framework (NIST AI RMF) is a guideline developed by the U.S. National Institute of Standards and Technology to help organizations identify, assess, and manage AI risks. It provides a structured approach to ensuring AI is trustworthy, secure, and fair.
Key components of the framework include:
- Govern – Establish AI governance policies, risk controls, and ethical standards.
- Map – Identify potential risks in AI models and how they may impact users.
- Measure – Evaluate AI risks using key metrics like bias, explainability, and security vulnerabilities.
- Manage – Implement risk mitigation strategies and continuous monitoring.
Organizations using AI for healthcare, finance, and defense rely on NIST AI RMF to align AI operations with best practices and regulations.
10. What Are the Biggest Risks of AI?
AI has transformed industries, but it also comes with significant risks, including:
- Bias & Discrimination – AI models can reinforce gender, racial, or economic biases if trained on unbalanced data.
- Security Threats – AI can be hacked, manipulated, or poisoned by adversarial attacks.
- Privacy Violations – AI systems handling personal data may breach GDPR, CCPA, or HIPAA regulations.
- Job Displacement – AI automation can replace jobs, leading to economic disruption.
- Lack of Explainability – Many AI models, especially deep learning, act as black boxes with unclear decision-making processes.
Managing these risks requires strong governance, bias detection, security measures, and human oversight.
11. What is AI Risk Assessment?
AI risk assessment is the process of analyzing AI models for potential risks before and after deployment. This ensures AI systems are safe, fair, and compliant with regulatory requirements.
Steps in an AI risk assessment:
- Identify risks – Assess AI models for bias, security flaws, and ethical concerns.
- Evaluate likelihood and impact – Use risk scoring models to rank AI risks from low to critical.
- Test AI outputs – Conduct bias audits, adversarial testing, and performance evaluations.
- Map risks to regulations – Ensure AI meets standards like GDPR, AI Act, and ISO 42001.
- Monitor continuously – Implement AI monitoring tools to track risks in real time.
Without proper AI risk assessments, companies risk deploying flawed AI that could cause harm or legal issues.
12. What Are the Main AI Security Risks?
AI security risks are growing as hackers develop new ways to exploit AI systems. The biggest threats include:
- Adversarial Attacks – Hackers manipulate AI inputs (e.g., modifying an image slightly so AI misidentifies it).
- Data Poisoning – Attackers inject malicious data into AI training sets to corrupt outputs.
- AI Model Theft – Competitors or cybercriminals steal proprietary AI models through reverse engineering.
- Deepfake & AI-generated Misinformation – AI can be used to generate fake videos, manipulate voices, and spread misinformation.
- Weak Authentication in AI Systems – Poor security in AI-powered chatbots or automation tools can expose sensitive company data.
To mitigate these threats, organizations must:
- Encrypt AI training data to prevent unauthorized access.
- Use adversarial defenses to protect AI from manipulation.
- Regularly audit AI security vulnerabilities with penetration testing.
Ignoring AI security risks leaves businesses vulnerable to data breaches, fraud, and compliance failures.
13. How Can Businesses Mitigate AI Risks?
AI risk mitigation involves reducing AI-related threats while ensuring AI models are fair, transparent, and secure. Some key strategies include:
✅ Bias & Fairness Mitigation
- Use diverse training datasets to prevent bias.
- Conduct regular AI audits to test for discrimination.
- Implement fairness-aware machine learning techniques.
✅ Security & Privacy Protection
- Encrypt AI models and implement strong access controls.
- Detect adversarial attacks before they manipulate AI decisions.
- Use anonymization techniques to protect sensitive data.
✅ Operational & Business Risk Controls
- Ensure human oversight for high-stakes AI decisions (e.g., hiring, lending).
- Establish incident response plans to handle AI failures.
- Continuously monitor AI model drift and accuracy over time.
Effective AI risk mitigation protects businesses from compliance violations, security threats, and reputational damage.
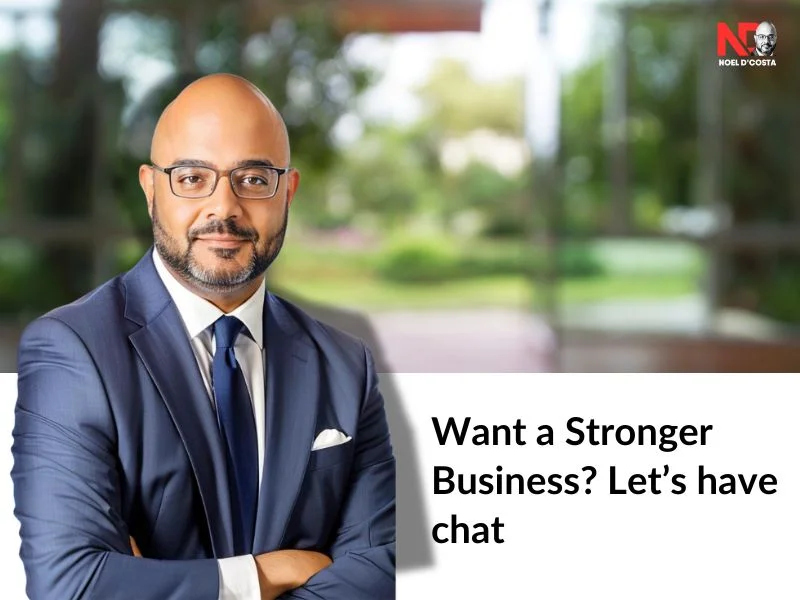