AI Governance Services for ERP, SAP, and Enterprise AI Deployments
AI governance services are becoming less of an optional investment and more of a necessary part of enterprise technology planning—especially when ERP systems, SAP platforms, and AI tools start intersecting. There’s a lot happening in that space. Companies are moving fast, integrating machine learning into business-critical workflows, but the oversight part tends to lag behind.
And that’s where it gets tricky.
You might already have AI running in your HR modules, or predictive models baked into supply chain forecasting. But what rules guide those systems? Who’s accountable if something goes wrong, or if results turn out biased? Sometimes, the answer is no one in particular. That’s a risk, and a gap AI governance aims to close.
AI governance services focus on helping enterprises define and enforce policies that manage how AI is built, tested, and used. Not just from a compliance angle, but to make sure these systems are dependable, explainable, and fair—at least to a practical degree.
Why AI Governance Services Matter?
When enterprises start integrating AI into ERP systems or SAP platforms, the risks shift. There is more complexity, more automation, and more potential for decisions to be made without direct human review.
That creates pressure—from both internal stakeholders and regulators.
- Enterprise Risk and Accountability in AI Systems
No one wants to be the one explaining a flawed model decision during an audit. But without clear AI governance structures, accountability becomes hard to track. AI models used in finance, procurement, or HR need oversight, especially when they influence real outcomes. - Regulatory Pressures on ERP and SAP-Driven AI
Compliance is tightening. Between the EU AI Act, industry guidelines, and internal policies, governance is now part of deployment strategy. - Failures of Unregulated AI in Core Business Systems
Misaligned models, biased results, or unexplained decisions can do real damage. Financially and reputationally. Some of that can be avoided with structured oversight.
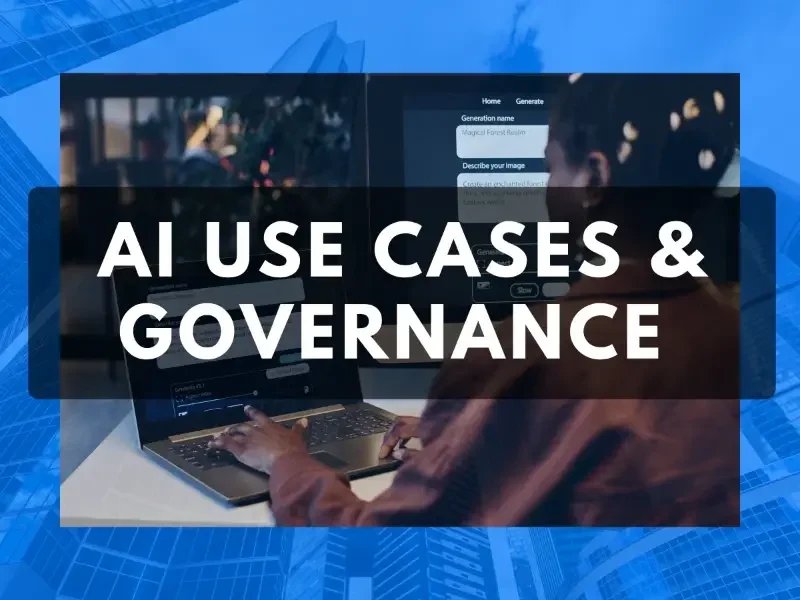
Core Components of Effective AI Governance Services
When people talk about AI governance services, it often sounds more complex than it really is. But in most enterprise environments—especially those built on SAP or ERP systems—it comes down to structure. Clear policies. Roles defined. Processes that do not just exist on paper but are used day to day. Without those, governance tends to drift, especially once AI moves from pilot to production.
Some companies try to retrofit governance after deployment. That usually leads to gaps, delays, and confusion. In our experience, it works better when governance is baked into how AI systems are designed, tested, and maintained. Not just for compliance, but for basic functionality and trust. Otherwise, small decisions can become large risks.
Policy Design and Oversight Structures
1. Policy Design and Oversight Structures
Effective AI governance services begin with solid policy frameworks. These are more than just guiding principles. They define who is responsible for decisions, how models are approved, and what oversight structures are in place to keep everything accountable.
- Defined roles across technical, legal, and business teams
- Model approval checkpoints and review workflows
- Documentation standards for versioning and decision logs
- Escalation protocols for flagged or high-risk AI systems
2. AI Model Accountability and Lifecycle Management
AI models need governance long after deployment. Lifecycle management ensures each model is continuously aligned with its intended purpose. Without it, even a strong model can drift or fail without warning.
- Ownership tracking from development through retirement
- Defined retraining triggers and thresholds for performance
- Scheduled review checkpoints with detailed audit logs
- Monitoring tools to detect data and behavior drift
3. Ethical and Legal Guardrails for Enterprise AI
Legal and ethical risks are often underestimated. AI governance services help identify areas where model behavior may cross regulatory or ethical boundaries—before those problems surface publicly.
- Fairness checks and bias impact assessments
- Pre-launch model risk and legal compliance review
- Data use policies aligned with jurisdictional rules
- Red lines for sensitive or high-impact use cases
4. AI Governance for ERP and SAP Platforms
ERP and SAP environments come with structured data and high accountability. When AI enters that ecosystem, governance must be tight and well-aligned with platform architecture and compliance models.
- Integration with SAP AI Core and Business AI tools
- Control layers around predictive analytics in SAP modules
- Governance embedded in change and release cycles
- Audit support aligned with ERP risk policies
5. Risk Assessment and Control Mechanisms
Without structured risk assessment, AI implementation can expose the business to compliance violations, data misuse, and operational errors. Controls must be both proactive and measurable.
- Standardized model risk classification frameworks
- Real-time control systems for key decision points
- Tools to assess third-party and open-source model risk
- Escalation matrices tied to business impact levels
6. Compliance and Regulatory Readiness
AI governance services also prepare organizations for audits and compliance checks. From the EU AI Act to NIST frameworks, aligning early helps reduce delays and avoid missteps later.
- Documentation standards to support regulatory audits
- Pre-mapped controls for major AI compliance frameworks
- Reporting tools for internal and external stakeholders
- Compliance tracking linked to ERP and GRC platforms
AI Model Accountability and Lifecycle Management
1. Model Ownership and Accountability
Every model should have a clear owner. Someone responsible for its intent, outputs, and performance over time. Without that, accountability gets blurry once models are deployed.
- Assign ownership at deployment stage
- Map responsibilities across technical and business functions
- Ensure continuity through model handovers
2. Model Versioning and Change Tracking
AI models change often—new data, code updates, feature tweaks. AI governance services ensure all of that is tracked. Not just the end result, but the process that led there.
- Use version control systems integrated with MLOps tools
- Maintain change logs tied to deployment events
- Tag updates to business outcomes or risk profiles
3. Retraining Triggers and Drift Detection
Data shifts. Business goals evolve. What worked last quarter may underperform today. Model retraining should not be reactive—it should be planned and based on clear thresholds.
- Define acceptable ranges for model drift
- Set retraining thresholds based on accuracy or risk
- Automate alerts when models deviate from expected behavior
4. Scheduled Performance Reviews
Governance is not a one-time check. Regular performance reviews help ensure models continue to meet their objectives and stay within compliance boundaries.
- Establish review intervals tied to business impact
- Compare current performance to baseline expectations
- Document findings and recommended actions
5. Audit-Ready Logging and Diagnostics
Audit trails are not just for compliance—they are practical. When models fail or outcomes are questioned, logs provide the evidence needed to diagnose and correct issues.
- Capture inputs, outputs, and decision pathways
- Enable log retrieval aligned with legal retention policies
- Support traceability down to data source level
6. End-of-Life and Model Retirement
Eventually, every model reaches its limit. AI governance services help define what that looks like and how retirement should be handled—without disruption to operations.
- Define criteria for model obsolescence
- Plan transitions to new models or manual processes
- Retain documentation for historical reference
Ethical and Legal Guardrails for Enterprise AI
1. Fairness Audits Before Deployment
Bias in AI often hides in plain sight. Fairness audits help uncover how a model might impact different groups before it's rolled out. Better to find issues early than explain them later.
- Run tests across gender, ethnicity, age, and other factors
- Use domain-specific fairness metrics, not just generic ones
- Document audit findings and outcomes in governance records
2. Data Usage Limits and Restrictions
Not all data is fair game. Some inputs, even if technically available, create legal or ethical risks. AI governance sets the boundaries for what data should never influence model behavior.
- Define disallowed features like race or health conditions
- Create approval workflows for sensitive data types
- Audit training datasets for hidden or proxy variables
3. Legal Reviews of Automated Decisions
Models that affect people—credit approvals, hiring, pricing—need a legal lens. Governance programs include formal review steps to ensure AI aligns with laws and policies.
- Review model outputs against anti-discrimination laws
- Validate automated decisions under sector-specific regulations
- Log legal sign-offs as part of governance workflows
4. Consent and Transparency Protocols
People affected by AI systems deserve to know when and how they are being evaluated. Clear communication builds trust and meets growing legal requirements.
- Ensure AI decisions are explainable in plain language
- Publish data use notices and obtain valid consent
- Provide opt-out mechanisms where appropriate
5. Human Oversight and Appeals Processes
Even the most accurate model can make a bad call. Governance should allow for human review and clear paths to challenge or reverse automated outcomes.
- Define which decisions require human approval
- Set response timelines for appeals or disputes
- Track override rates to identify weak model logic
6. Ethical Risk Assessments for New Use Cases
Not every application of AI is appropriate. Before expanding into new areas, ethical reviews help determine whether the use case crosses a line—legally, socially, or reputationally.
- Score use cases on potential for harm, bias, or misuse
- Review against internal ethics guidelines and public expectations
- Document decisions for internal and external accountability
How Can I Help You?
With over two decades in SAP and digital transformation, I’ve seen projects from kickoff to go-live—and the messy middle no one talks about. Sometimes I lead from the start. Other times, I’m brought in to steady the ship when things go sideways.
Either way, my role is the same: connect what the business really needs with what the system can actually deliver. No jargon. No fluff. What you’ll find here isn’t theory—it’s shaped by years in the field, solving real problems under real pressure.
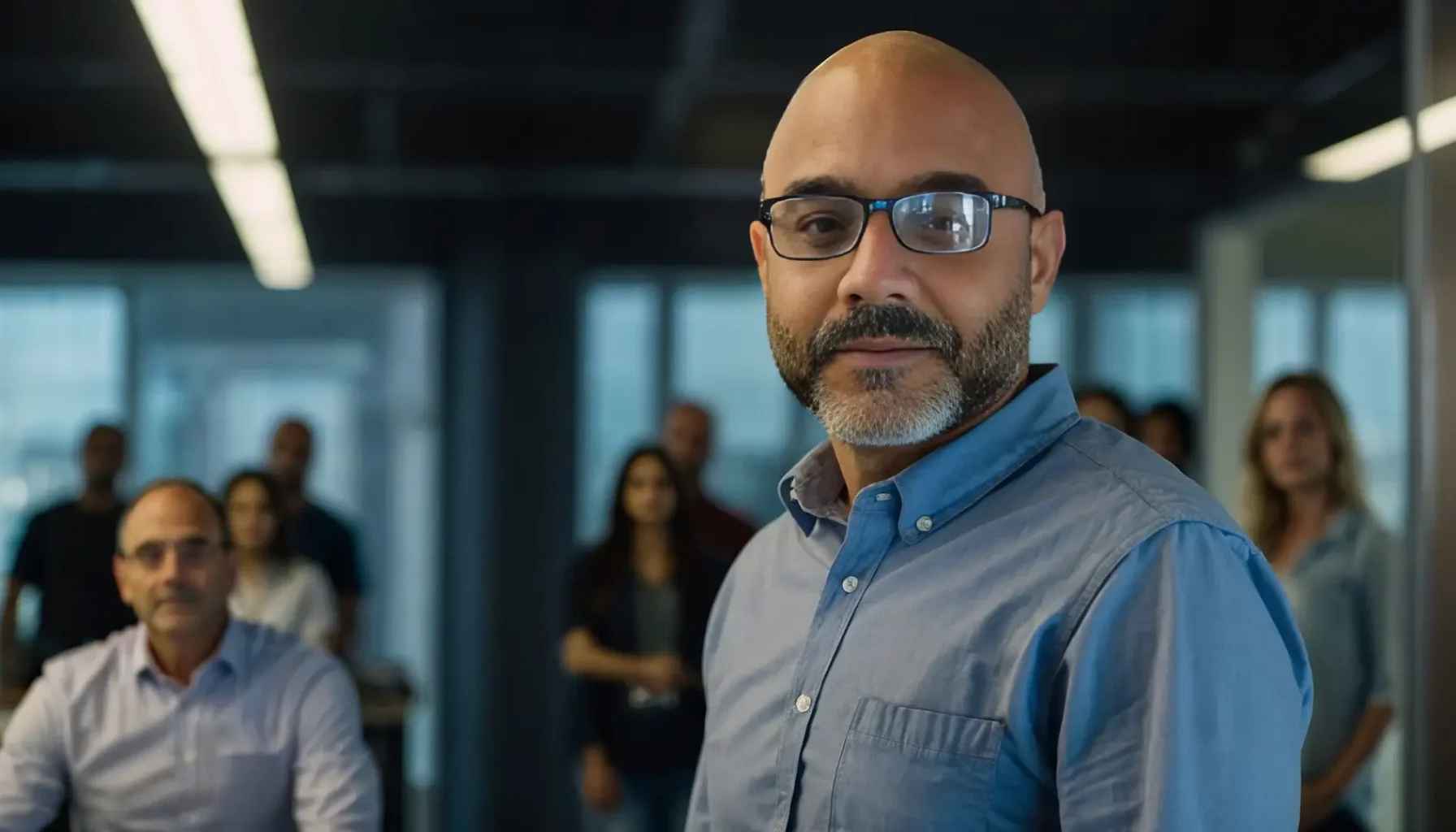
AI Governance Services for ERP and SAP Environments
AI governance inside ERP and SAP environments can feel a bit abstract at first. It sounds like a policy thing, or maybe something technical teams handle behind the scenes. But once machine learning starts influencing financial decisions or workforce planning, the need for structure becomes obvious. You start noticing where accountability blurs.
This is where formal AI governance services come in. They help build a system for control, one that stays aligned with the way ERP and SAP actually function in day-to-day operations.
Some areas where governance shows up:
Risk scoring inside SAP modules
Validation of model outcomes
Ongoing monitoring tied to business workflows
Core AI Governance Services for ERP and SAP Environments
1. AI Risk Profiling for SAP Business Modules
Each SAP module carries a unique risk when AI is applied. Finance, HR, procurement—all respond differently to automation. AI governance maps and ranks these risks to prioritize control implementation.
- Perform module-specific AI risk assessments
- Classify models by impact and data sensitivity
- Link risk ratings to internal control requirements
2. Embedded Risk Controls in SAP Processes
Risk management works better when it is part of the workflow. AI governance embeds model checkpoints within SAP processes, not as side tasks, but directly in the business flow.
- Configure alerts for model anomalies within SAP
- Enforce review gates in SAP transactional logic
- Align model risk tolerance with GRC policies
3. Financial AI Model Testing in SAP
Models used in forecasting, revenue, and credit analysis require high validation precision. SAP financial data offers the historical baseline needed to validate predictions with real-world impact.
- Cross-validate outputs with SAP FI and CO data
- Check for volatility across fiscal periods
- Audit assumptions used in financial modeling
4. HR and Operational Model Checks
AI in HR or operations affects people and processes. Governance must cover not just accuracy, but fairness and alignment to policy—especially in SuccessFactors or supply chain modules.
- Test model bias across employee groups
- Validate resource planning models against service KPIs
- Monitor model updates tied to workforce impact
5. Governance Controls in SAP AI Core
SAP AI Core enables flexible model deployment, but governance keeps those models compliant and monitored. Integration helps track behavior and flag violations before they reach production scale.
- Incorporate automated logging for deployed models
- Use SAP AI Launchpad roles to restrict access
- Tie model performance to compliance KPIs
6. ERP Extension and Third-Party Model Governance
AI-enabled ERP extensions often come from third parties. Governance ensures those models follow the same enterprise rules—especially around data access, retention, and explainability.
- Run vendor model audits and documentation reviews
- Verify extension compliance with AI usage policies
- Integrate external models into internal audit tracking
AI Governance Framework Development
Building an AI governance framework sounds straightforward at first—until you start figuring out who actually owns what, and where policies fit into everything else already running. The hard part is not drafting documents. It is making them usable across real systems, with real teams who already have too much on their plates.
In practice, it helps to start with structure. Just enough to make decisions clearer. From there, the pieces begin to connect:
Roles with actual accountability
Policies that reflect how systems actually work
Integration points that do not feel bolted on
It is more alignment than overhaul. Most of the time.
AI Governance Framework Development Services
1. Role Mapping Across AI Governance Functions
Effective AI governance starts with clear roles. Everyone involved—from data scientists to compliance leads—should know what they’re accountable for, and when to escalate.
- Assign ownership at each stage of the AI lifecycle
- Differentiate roles between oversight and execution
- Document responsibilities in governance playbooks
2. Escalation Protocols for AI Model Risks
When something goes wrong—or seems off—teams need to know where to go. Escalation paths are not just for emergencies. They are part of daily governance hygiene.
- Define model-specific escalation thresholds
- Route incidents through existing enterprise service desks
- Log and audit responses for future analysis
3. Aligning Governance Policies with Technical Stack
Policies are only effective if they reflect the systems in use. AI governance frameworks need to fit the architecture—ERP systems, cloud platforms, data pipelines—without forcing rework.
- Map policy enforcement points to system workflows
- Use policy templates customized for ERP and SAP setups
- Ensure data flow restrictions match security zones
4. Model Governance Embedded in Enterprise Platforms
AI models often touch multiple systems. Governance policies must be present wherever models operate—not just at deployment. That includes infrastructure and integration layers.
- Tie policies to containerization and API layers
- Standardize access control across model endpoints
- Validate compliance within orchestration pipelines
5. Integrating Governance with GRC Systems
Many enterprises already use GRC platforms for risk and compliance. The right AI governance approach feeds directly into those tools—no parallel process needed.
- Map model risks to enterprise risk taxonomies
- Use existing GRC workflows for approvals and tracking
- Auto-log governance activity for audit readiness
6. Connecting Governance to DevOps and MLOps
Governance does not have to slow development. When baked into DevOps or MLOps, it becomes part of the delivery pipeline—quietly enforcing quality and control.
- Trigger model reviews during CI/CD stages
- Apply policy checks to version control and builds
- Log governance checkpoints directly into model registries
Risk Assessment and Control Implementation
AI risks are not always loud or obvious. Sometimes they creep in through minor changes—a shift in data quality, a model retrained without much review, or just a missed checkpoint. It happens. Especially in systems like ERP or SAP, where things run quietly in the background until, well, they don’t.
That is why structured risk assessment matters. You are not solving everything at once. You are building layers of visibility.
Some of that involves:
Tracking where models operate
Classifying risks based on impact
Making sure there is a plan for when things go off track
It is not overengineering. It is just preparation.
Risk Assessment and Control Implementation Services
1. Mapping AI Risk Across Business Functions
Before risks can be managed, they need to be understood. That starts with identifying where AI models sit, what they impact, and how they align with business-critical functions.
- Inventory all AI models tied to ERP workflows
- Tag models based on sensitivity and decision impact
- Classify risks into categories like bias, performance, or data exposure
2. Risk Scoring and Prioritization Methods
Not every risk deserves the same level of control. Prioritization helps allocate effort where it matters most. Usually that means looking at impact, likelihood, and traceability.
- Apply weighted scoring to model risks
- Use a heatmap to align risk with control strength
- Link high-priority models to escalation paths
3. Embedding Controls Within ERP Workflows
Controls need to live where the work happens. That means embedding checkpoints into the ERP system, where models actually influence business decisions.
- Insert validation steps in SAP and ERP logic flows
- Configure role-based approvals for model actions
- Align controls with data access and transaction layers
4. Control Testing and Enforcement Models
Designing controls is one thing. Testing whether they work—and hold up under pressure—is what makes governance real. You will need repeatability and reporting.
- Simulate edge cases to test control behavior
- Document control failures and follow-up actions
- Log enforcement outcomes to feed back into governance reviews
5. Monitoring Model Behavior in Production
Once AI is live, passive oversight will not be enough. Active monitoring lets teams catch issues early—before they spiral into operational problems.
- Track model drift, performance, and exceptions
- Monitor changes in input data patterns or outcomes
- Feed monitoring insights into performance dashboards
6. Incident Detection and Response Playbooks
No system is perfect. The question is how fast you catch issues—and what you do next. Response protocols help take the guesswork out of urgent situations.
- Create predefined response plans by incident type
- Route alerts to the right team with clear roles
- Document incidents for audit and policy updates
AI Governance Services Blog
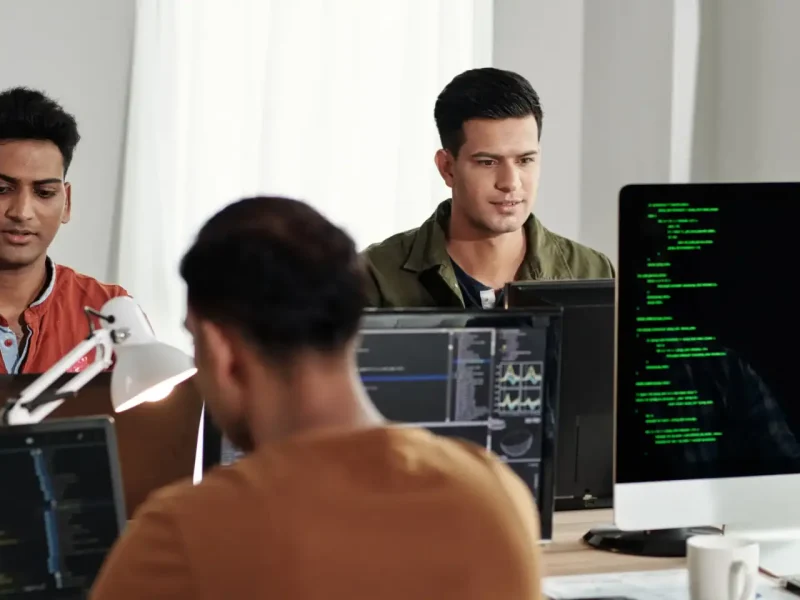
Regulatory Compliance and Audit Readiness
Regulatory compliance in AI is shifting faster than many teams can comfortably keep up with. The EU AI Act is a big one, but there is also NIST’s AI Risk Management Framework and several sector-specific guidelines. Trying to align with all of them can feel a bit overwhelming at first.
The key, really, is structure. Governance frameworks that support explainability, documentation, and traceability are what make compliance repeatable. Without that, audits tend to become a scramble.
A few practical areas to focus on:
Maintain consistent documentation during the entire model lifecycle
Ensure outputs can be explained in non-technical terms
Automate logging and tracking for audit readiness
You do not need perfection. You need visibility and a trail that holds.
Responsible AI Deployment Services
Audit readiness with AI is not just about storing logs or ticking compliance boxes. It has more to do with making sure your systems can explain themselves—clearly, consistently, and without too much friction. That part often gets missed.
You might think the tech team has it covered, and maybe they do, but when auditors show up asking why a model did what it did last quarter, silence is not an option.
What helps:
Documenting key model decisions as they happen
Linking outcomes to actual data inputs
Keeping audit trails automated, not manual
It sounds like overhead, but it saves time later. Every time.
1. Functional Testing for ERP-Embedded AI
Before deployment, models should be tested in real-world business contexts—not just sandbox environments. ERP-integrated AI often behaves differently once exposed to live data and workflows.
- Test AI outputs within ERP transaction flows
- Use historical SAP data for scenario validation
- Document edge cases and exceptions clearly
2. Model Performance and Stress Testing
AI under load can behave differently. Testing how models hold up during peak business cycles—especially in supply chain or finance—is critical before go-live.
- Simulate high-volume transaction scenarios
- Validate model response time under load
- Benchmark performance against defined SLAs
3. Fairness Audits in People-Centric Models
When AI is used in HR or financial decision-making, fairness matters. Governance services help identify bias and ensure models meet ethical expectations before production.
- Audit training data for representation gaps
- Use demographic filters in model validation
- Log bias test results for governance review
4. Mitigation Strategies for Detected Bias
Finding bias is not enough. There must be clear steps for how to address it, with governance teams involved in approving any mitigations applied before deployment.
- Retrain models with adjusted sampling techniques
- Remove or limit use of proxy features
- Review mitigation outcomes with legal and HR leads
5. Approval Chains for High-Risk Models
Not all models require the same level of review. High-impact use cases—finance, workforce, compliance—need formal workflows with approvals before deployment into ERP systems.
- Define model risk categories and required sign-offs
- Route high-risk models through cross-functional review
- Log decisions in compliance and audit systems
6. Deployment Readiness Checks
Before any model goes live, a final checkpoint ensures everything is in place—testing, approvals, risk classification, and rollback options if needed.
- Run a checklist review with governance and IT teams
- Confirm monitoring tools are active and alerting
- Establish rollback or override procedures post-launch
Content developed by Noel D’Costa | https://noeldcosta.com
Frequently Asked Questions
A lot of clients tend to circle around the same questions when they’re first considering an SAP implementation.
Maybe you’ve had a few of them yourself—how long it really takes, what it might cost, or what kind of support is needed once the system goes live. Fair questions.
So instead of leaving you guessing, we’ve pulled together clear, honest answers to help you get a better sense of what to expect, and where the tricky parts usually show up.
1. What are AI Governance Services, exactly?
They are structured programs that help organizations manage how AI systems are built, deployed, and monitored. Think of it as a mix of policy, oversight, and technical checks. It is not only for compliance—it is also about reducing risk and improving outcomes over time.
2. Do I need AI governance if we only use basic models?
Maybe. Even smaller models can create problems if they are used in finance, HR, or anything customer-facing. Risk tends to scale with exposure, not just model complexity. So yes, simple models sometimes need governance too.
3. How does AI governance fit into ERP and SAP systems?
It works best when it is embedded—not added after the fact. That might mean adding approval steps in SAP workflows, testing models inside ERP transactions, or monitoring output directly from business systems. It depends on your setup.
4. Who is responsible for AI governance in a typical company?
That varies. Some assign it to IT. Others put it under risk, legal, or compliance. Ideally, it is shared. Data scientists, business leads, compliance teams—they all play a role. Without clear ownership, it tends to fall through the cracks.
5. What is the first step toward implementing AI governance?
Start with a model inventory. Just knowing what AI you are using, where, and why is more valuable than it sounds. From there, you can assess risk, draft policies, and figure out what kind of controls make sense.
6. How often should models be reviewed?
It depends on the risk. Some need quarterly reviews. Others might go longer. But even if nothing changes, a review process helps catch small issues before they become real ones.
7. How does this relate to compliance requirements like the EU AI Act?
Directly. Many new regulations are now requiring explainability, documentation, fairness, and auditability. AI governance services help you put those elements in place so you are not reacting when the rules change.
8. What happens if a model fails or causes an issue?
If governance is in place, there should be an audit trail, a rollback plan, and a process for review. If not, teams usually scramble to figure out what went wrong and why. That delay alone can be damaging.
9. Can AI governance slow down innovation?
Not if it is designed properly. In fact, many teams find that with the right governance structure, they move faster—because reviews are clear, decisions are logged, and risks are addressed early. It reduces rework later.
10. Is this something we can build internally, or do we need external help?
Some do it in-house. Others bring in support to get the structure right from the start. It depends on internal expertise. But even if you build it yourself, a second set of eyes can help uncover blind spots.